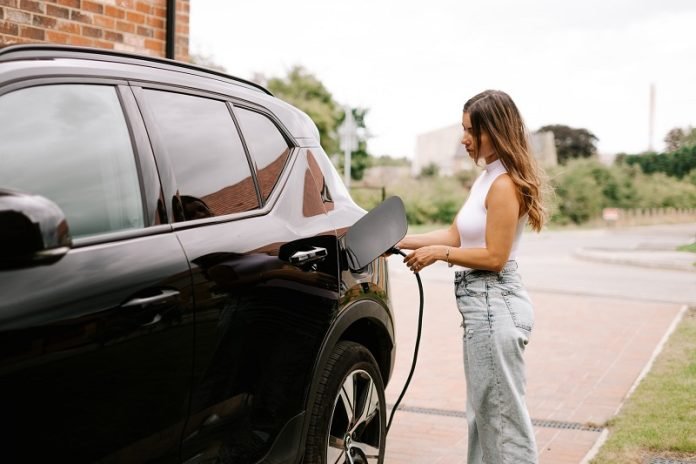
Electric vehicles (EVs) have gained popularity worldwide, thanks to the impressive capabilities of lithium-ion batteries.
However, the safe and efficient recycling of retired EV batteries has become a pressing concern.
In an exciting development, a research team led by Dr. Lin Mingqiang at the Fujian Institute of Research on the Structure of Matter, Chinese Academy of Sciences, has proposed a groundbreaking method for screening retired batteries.
Their study, published in Engineering Applications of Artificial Intelligence, introduces an innovative approach that combines advanced data analysis techniques to ensure effective battery sorting.
Let’s delve into this remarkable solution that could revolutionize battery recycling.
With the increasing adoption of electric vehicles, the proper handling and recycling of retired EV batteries have become critical issues.
Finding an efficient and accurate method to assess the condition of these batteries is essential for their safe disposal or potential reuse.
To address this challenge, Dr. Lin Mingqiang’s research team employed a unique approach. They started by simplifying the battery data using a technique called segmented aggregation approximation.
This process reduced the data’s complexity while retaining its crucial information, making it easier to analyze and process.
Next, the researchers transformed the simplified battery data into two-dimensional images using a mathematical tool called the Gramian Matrix (GM).
By utilizing a method called Gramian angular difference fields (GADF), they encoded the battery’s charging curve into the GM. This encoding technique eliminated unnecessary information, reduced the impact of irregular data patterns, and minimized noise interference.
To classify and identify retired batteries accurately, the researchers employed a powerful image classification network called ConvNeXt. This network used an optimizer called Adaptive Moment Estimation Weight Decay (AdamW) to fine-tune its parameters and achieve optimal performance.
By continuously updating and optimizing these parameters during training, the network learned to make accurate predictions based on input data.
The research team compared their GADF-based method with traditional approaches and evaluated different image classification networks.
They discovered that using GADF as input for the ConvNeXt network significantly improved the accuracy of retired battery screening. This breakthrough allows for reliable and data-driven analysis, reducing the need for manual selection features.
While this study has already revolutionized battery recycling practices, future research will explore screening strategies based on partial voltage and expand the dataset for broader validation of the model’s performance.
In the quest for more sustainable practices, the recycling of retired electric vehicle batteries is a crucial step.
The innovative method proposed by Dr. Lin Mingqiang’s team offers a data-driven approach to efficiently and accurately screen these batteries.
By utilizing advanced techniques like GADF and ConvNeXt, the researchers have paved the way for improved battery recycling processes.
This breakthrough holds the potential to make battery disposal safer and more eco-friendly, contributing to a greener future.