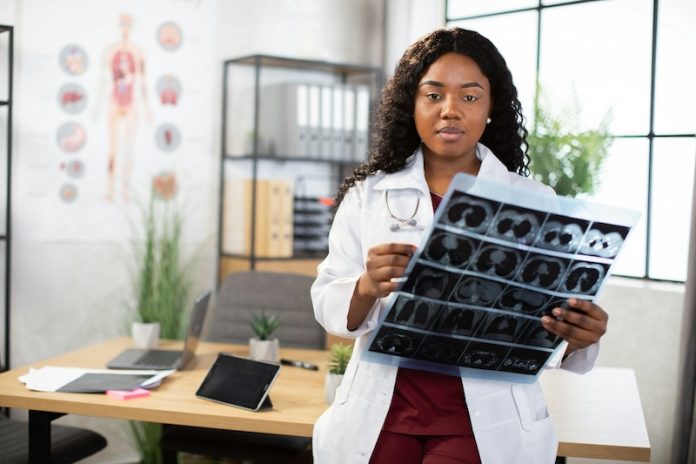
A new study published in Nature Machine Intelligence explores how artificial intelligence (AI) can help doctors analyze medical images more efficiently. Researchers from Monash University’s Engineering and IT faculties have developed a new AI approach that improves how medical images are labeled, reducing the need for time-consuming manual work by doctors.
Medical imaging, such as X-rays, MRIs, and CT scans, plays a key role in diagnosing diseases. Radiologists and other medical experts examine these images to detect issues like tumors, infections, or broken bones. To train AI systems for medical use, researchers typically need large collections of images that have been manually labeled by experts.
These labels highlight areas of concern, making it easier for AI to learn and recognize similar patterns in new scans. However, creating these labeled datasets is a slow and difficult process.
It requires highly skilled professionals and can take weeks or months to complete. As a result, there is often a shortage of labeled medical images, limiting the effectiveness of AI in medical diagnosis.
To overcome this challenge, the Monash University team designed an AI system that learns from both labeled and unlabeled images. Ph.D. candidate Himashi Peiris, one of the researchers involved, explained how the system works.
The AI has two parts that compete against each other, similar to a game. One part tries to label medical images just like a human radiologist would, while the other part checks the quality of those labels by comparing them to the limited expert-labeled images available.
Through this process, the AI improves its accuracy over time, even when it has only a small number of labeled images to learn from.
Traditionally, AI models need thousands of carefully labeled images to work well. The Monash researchers, however, have developed an algorithm that allows the AI to learn from both labeled and unlabeled data, helping it make better predictions with much less human input.
This approach is known as semi-supervised learning, meaning the AI does not rely entirely on human supervision but still improves its performance through a mix of expert-labeled and unlabeled data.
The team tested their method using three publicly available medical image datasets. Even when only 10% of the images were labeled, their AI model improved accuracy by an average of 3% compared to the latest AI techniques used under the same conditions.
This improvement may seem small, but in medical diagnostics, even a slight increase in accuracy can lead to better patient outcomes and more reliable medical decisions.
Peiris described the research as a major step forward in medical AI. “Our method outperforms previous approaches by achieving high accuracy with fewer labeled images. This means AI can provide better support for doctors, helping them make more confident and accurate diagnoses.”
The researchers plan to continue their work by testing their AI on different types of medical images and developing a complete system that radiologists can use in hospitals and clinics. The ultimate goal is to create an AI-powered tool that can assist doctors in analyzing scans quickly and accurately, reducing workload and improving patient care.
Analysis of the Study
This research highlights an important advancement in medical AI by reducing reliance on expert-labeled images. The findings show that AI can still achieve high accuracy even with limited data, which could make medical AI more practical and widely used.
The system’s ability to refine its own predictions and learn from both labeled and unlabeled images is a significant improvement over older methods that required extensive human input.
However, while the results are promising, AI systems in healthcare still need rigorous testing before they can be trusted for real-world use. Accuracy in medical diagnostics is critical, as errors could lead to misdiagnosis or incorrect treatment.
Future studies should focus on testing the AI with different diseases, imaging techniques, and patient populations to ensure reliability across various medical conditions.
If successfully implemented, this AI model could save doctors time, reduce human error, and improve access to quality healthcare, particularly in regions where trained radiologists are in short supply. By continuing to refine and expand this technology, researchers can help bring AI-assisted medical imaging closer to everyday clinical practice.
If you care about medicine, please read studies that vitamin D could help lower the risk of autoimmune diseases, and drug for inflammation may stop spread of cancer.
For more health information, please see recent studies about which drug can harm your liver most, and results showing this drug can give your immune system a double boost against cancer
The research findings can be found in Nature Machine Intelligence.
Copyright © 2025 Knowridge Science Report. All rights reserved.