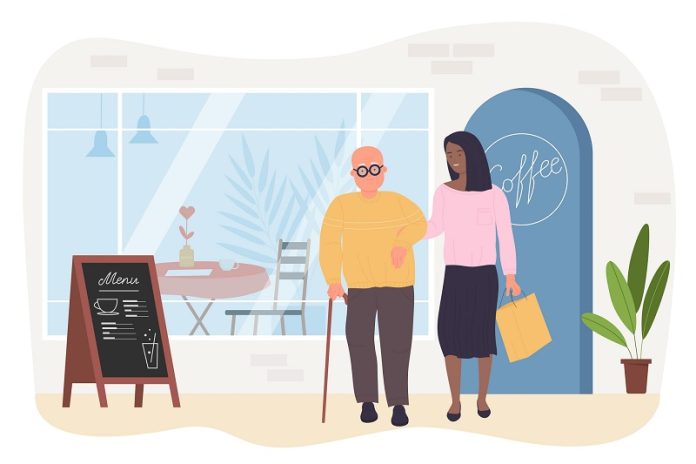
Scientists from Cambridge have created an AI tool that can predict whether people showing early signs of dementia will stay stable or develop Alzheimer’s disease.
This tool works accurately in four out of five cases.
This new method could help reduce the need for expensive and invasive tests while allowing for early treatments, which might be more effective during the early stages of the disease.
Dementia is a major global health issue, affecting over 55 million people worldwide and costing an estimated $820 billion each year. The number of cases is expected to nearly triple in the next 50 years.
Alzheimer’s disease is the main cause of dementia, making up 60-80% of cases. Early detection is crucial because treatments are more effective at this stage.
However, early diagnosis is often inaccurate without using costly and invasive tests like PET scans or lumbar punctures, which are not always available.
Due to the limitations of current methods, many patients are misdiagnosed or diagnosed too late for treatments to be effective. To address this, a team from the University of Cambridge’s Department of Psychology has developed a machine learning model.
This model can predict whether a person with mild memory and thinking problems will develop Alzheimer’s disease and how quickly it might happen. Their research, published in eClinicalMedicine, shows this model is more accurate than current clinical diagnostic tools.
The researchers used data that is routinely collected, non-invasive, and low-cost, such as cognitive tests and MRI scans.
They gathered data from over 400 individuals in a research group in the US and then tested the model with real-world patient data from another 600 US participants. They also used data from 900 people from memory clinics in the UK and Singapore.
The AI tool could distinguish between people with mild cognitive impairment who would remain stable and those who would develop Alzheimer’s within three years.
It correctly identified individuals who would develop Alzheimer’s in 82% of cases and those who wouldn’t in 81% of cases. This is significantly more accurate than current methods, which rely on clinical markers or diagnoses.
The model also allowed the researchers to classify people with Alzheimer’s disease into three groups based on their first visit to the memory clinic:
those whose symptoms would remain stable (about 50% of participants), those who would progress slowly (about 35%), and those who would progress rapidly (about 15%). These predictions were confirmed when looking at follow-up data over six years.
This classification is crucial because it can help identify people early enough to benefit from new treatments. It also highlights those who need close monitoring due to rapid progression.
Furthermore, for the 50% of people with memory loss who remain stable, their symptoms might be due to other causes like anxiety or depression, and they can be directed to appropriate care.
Professor Zoe Kourtzi from the University of Cambridge’s Department of Psychology said that the tool, despite using only data from cognitive tests and MRI scans, is much more sensitive than current approaches.
This sensitivity can improve patient well-being by identifying those who need close care and reducing anxiety for those who will remain stable. It can also help reduce the need for unnecessary tests, saving resources.
The researchers tested the algorithm with data from research groups and validated it with data from nearly 900 individuals from memory clinics in the UK and Singapore.
In the UK, patients were recruited through a study led by Dr. Timothy Rittman at Cambridge University Hospitals NHS Trust and Cambridgeshire and Peterborough NHS Foundation Trusts (CPFT).
Dr. Ben Underwood, a consultant psychiatrist at CPFT and assistant professor at the University of Cambridge, noted that memory problems are common with aging, and uncertainty about dementia can cause significant worry.
Reducing this uncertainty with existing information is exciting and important, especially as new treatments emerge.
Professor Kourtzi emphasized that AI models are only as good as the data they are trained on. Their model was trained and tested on data from both research cohorts and real-world patients, showing it can be applied in clinical settings.
The team hopes to extend their model to other forms of dementia, such as vascular dementia and frontotemporal dementia, using various types of data, including blood tests.
The team’s goal is to scale up their AI tool to help clinicians diagnose and treat dementia at the earliest possible stage. This tool could also match the right patients to clinical trials, speeding up the discovery of new treatments.
For more information about dementia, please see recent studies about brain food: nourishing your mind to outsmart dementia and results showing that re-evaluating the role of diet in dementia risk.
For more information about brain health, please see recent studies about the power of healthy fats for brain health and results showing that Mediterranean diet may preserve brain volume in older adults.
The research findings can be found in eClinicalMedicine.
Copyright © 2024 Knowridge Science Report. All rights reserved.