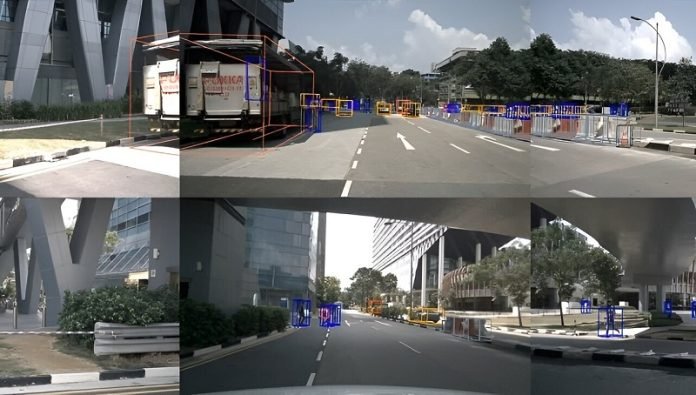
Researchers at the University of Toronto Institute for Aerospace Studies (UTIAS) have developed two innovative tools to improve the safety and reliability of self-driving cars.
These tools enhance the reasoning abilities of robotic systems, helping them better track the position and movement of objects like vehicles, pedestrians, and cyclists in busy areas.
The first tool, called Sliding Window Tracker (SWTrack), was created by Sandro Papais, a Ph.D. student, along with Robert Ren, an engineering science student, and Professor Steven Waslander, the director of UTIAS’s Toronto Robotics and AI Laboratory.
This tool uses extra information over time to prevent missing objects, making the tracking process more accurate.
Their findings were presented at the 2024 International Conference on Robotics and Automation in Yokohama, Japan, and are available on the preprint server arXiv.
Multi-object tracking involves collecting information from computer vision sensors, like 2D camera images and 3D LIDAR scans, which is then filtered ten times per second to predict the future movement of objects.
This helps self-driving cars make better decisions about their surroundings.
“Once processed, the robot can understand its environment,” Papais explains. “For instance, it can detect a person crossing the street or a cyclist changing lanes.”
The SWTrack tool looks further back in time, up to five seconds, to link current detections with past objects. This helps the robot better understand and predict movements. The team tested their tool using data from nuScenes, a public dataset of autonomous driving vehicles.
They found that the longer the temporal window, the better the tracking performance, up to a five-second limit, beyond which the computation time slowed down.
Papais is excited about the potential of this approach and plans to apply it to other areas of robotics. “This is just the beginning,” he says. “We aim to improve robot memory and extend it to other robotic tasks.”
The second tool, UncertaintyTrack, was developed by master’s student Chang Won (John) Lee and Professor Waslander. This tool uses probabilistic object detection to estimate the uncertainty of object detection, which is crucial for safety-critical tasks.
It helps the system know when its predictions might be wrong, such as in low-light conditions or when objects are heavily occluded.
Lee worked on this tool during his undergraduate thesis and is now researching visual anomaly detection for the Canadarm3, a robotic arm for the lunar Gateway outpost.
Professor Waslander highlights the importance of these advancements: “We need AI methods that understand the persistence of objects over time and are aware of their limitations. Our research aims to achieve this.”
By enhancing object tracking and reasoning abilities, these new tools could significantly improve the safety and reliability of self-driving cars, bringing us closer to a future where autonomous vehicles are a common sight on our roads.