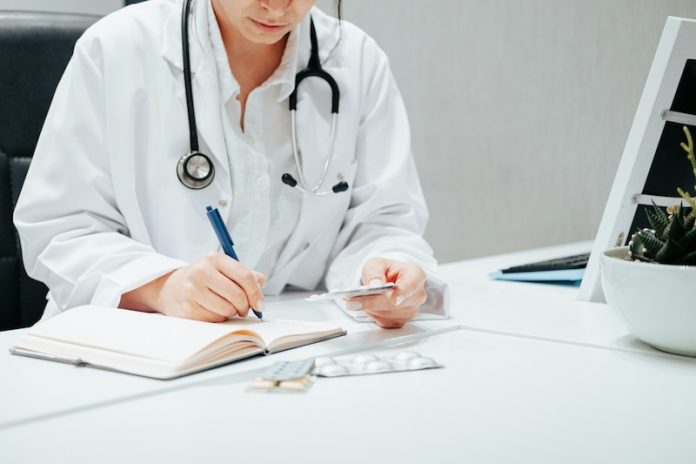
Researchers from UCLA Health have developed a groundbreaking machine learning tool, PheNet, that could potentially revolutionize the diagnosis of rare diseases like common variable immunodeficiency (CVID).
Published in Science Translational Medicine, the study demonstrates that PheNet may be able to identify patients with rare, undiagnosed diseases years before traditional methods would.
CVID is a complex disorder that often goes undiagnosed for years due to its rarity and the variability of symptoms among individuals. The condition is characterized by an immune deficiency that can lead to increased susceptibility to infections and other health complications.
Dr. Manish Butte, a key figure in the research and a professor at UCLA, points out that the delayed diagnosis of rare diseases like CVID can cause unnecessary testing, progressive illness, and significant psychological and financial stress for patients.
The traditional method of diagnosing CVID can be cumbersome, as symptoms overlap with many other conditions and can vary widely from person to person.
Moreover, because CVID can be driven by changes in over 60 different genes, there is no single genetic test that can definitively diagnose the disorder.
PheNet offers a solution by using machine learning to analyze patterns in electronic health records (EHRs).
It identifies phenotypic patterns — the observable characteristics of a disease — from verified cases of CVID and uses this information to rank patients according to their likelihood of having the disease.
This method allows for the potential early detection and referral of patients who might otherwise remain undiagnosed for extended periods.
The effectiveness of PheNet was highlighted by the research team’s findings that dozens of patients could have been diagnosed one to four years earlier than they were, using traditional diagnostic methods.
This early intervention could significantly reduce healthcare costs and improve health outcomes for patients.
Dr. Butte and Dr. Bogdan Pasaniuc, co-senior authors of the study, further validated PheNet using over 6 million patient records from multiple medical systems, including the University of California Data Warehouse and Vanderbilt Medical Center.
Their work has initiated a new collaboration with immunology clinics across University of California campuses to refine and expand the application of PheNet.
PheNet’s potential goes beyond CVID. The researchers believe that this AI tool could expedite the diagnosis of a range of rare diseases, offering new hope for patients who suffer from conditions that are currently difficult to diagnose.
This innovation comes at a crucial time, addressing the broader issue of healthcare inefficiency where different specialists might see only parts of a patient’s health puzzle without being able to see the bigger picture.
Dr. Ruth Johnson, lead author of the study and a fellow at Harvard Medical School, emphasized the broader implications of delayed diagnoses, including increased infections, higher medical costs, and significant personal and economic burdens.
In conclusion, the UCLA team’s development of PheNet is a promising step forward in the use of artificial intelligence in healthcare, providing a more streamlined, accurate, and cost-effective approach to diagnosing and managing rare diseases.
If you care about nutrition, please read studies about why vitamin K is so important for older people, and this snack food may harm your heart rhythm.
For more information about nutrition, please see recent studies about vitamin that may protect you from type 2 diabetes, and results showing this common chemical in food may harm your blood pressure.
The research findings can be found in Science Translational Medicine.
Copyright © 2024 Knowridge Science Report. All rights reserved.