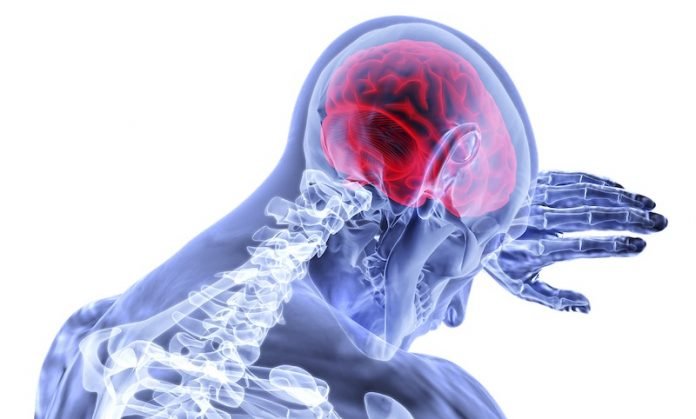
In a new study, UC San Francisco and UC Berkeley researchers found an algorithm did better than two out of four expert radiologists at finding tiny brain hemorrhages in head scans.
This advance one day may help doctors treat patients with traumatic brain injuries (TBI), strokes and aneurysms.
The continued increase in diagnostic imaging studies, including 3-D imaging studies such as computed tomography (CT), means that radiologists are looking at thousands of images each day, searching for tiny abnormalities that can signal life-threatening emergencies.
The number of images from each brain scan can be so large that on a busy day, radiologists may opt to scroll through some large 3-D stacks of images using mice with frictionless wheels, almost like viewing a movie.
But it could be much more efficient—and potentially more accurate—if AI technology could pick out the images with significant abnormalities, so radiologists could examine them more closely.
The algorithm the team developed took just one second to determine whether an entire head scan contained any signs of hemorrhage.
It also traced the detailed outlines of the abnormalities it found—demonstrating their location within the brain’s three-dimensional structure.
Some spots may be on the order of 100 pixels in size, in a 3-D stack of images containing over a million of them, and even expert radiologists sometimes miss them, with potentially grave consequences.
The new study made use of a type of deep learning known as a fully convolutional neural network, or FCN, which trains algorithms on a relatively small number of images, in this case, 4,396 CT exams.
But the training images used by the researchers were packed with information because each small abnormality was manually delineated at the pixel level.
The richness of this data—along with other steps that prevented the model from misinterpreting random variations or “noise” as meaningful—created an extremely accurate algorithm.
The algorithm could found some small abnormalities that the experts missed. It also noted their location within the brain, and classified them according to subtype, information that physicians need to determine the best treatment.
And the algorithm provided all of this information with an acceptable level of false positives—minimizing the amount of time that physicians would need to spend reviewing its results.
The radiology experts say the algorithm’s ability to find very small abnormalities and demonstrate their location in the brain was a substantial advance.
The authors are now applying the algorithm to CT scans from trauma centers across the country.
They say that given a large number of people who suffer from traumatic brain injury every day and are rushed to the emergency department, this has very big clinical importance.
One author of the study is Esther Yuh, MD, Ph.D., associate professor of radiology at UCSF.
The study is published in PNAS.
Copyright © 2019 Knowridge Science Report. All rights reserved.