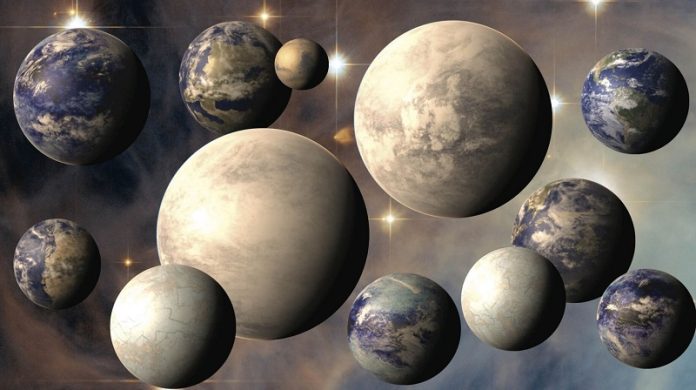
Modern astronomy would struggle without AI and machine learning (ML), which have become indispensable tools.
They alone have the capability to manage and work with the vast amounts of data that modern telescopes generate.
ML can sift through large datasets, seeking specified patterns that would take humans far longer to find.
The search for biosignatures on Earth-like exoplanets is a critical part of contemporary astronomy, and ML can play a big role in it.
Since exoplanets are so distant, astronomers pay close attention to the ones that allow transmission spectroscopy. When starlight passes through a planet’s atmosphere, spectroscopy can split the light into different wavelengths. Astronomers then examine the light for the telltale signs of particular molecules.
However, chemical biosignatures in exoplanet atmospheres are tricky because natural abiogenic processes can generate some of the same signatures.
Though the method is powerful, it faces some challenges. Stellar activity like starspots and flares can pollute the signal, and the light from the atmosphere can be very weak compared to the star’s light. If there are clouds or haze in the exoplanet’s atmosphere, that can make it difficult to detect molecular absorption lines in the spectroscopic data.
Rayleigh scattering adds to the challenge, and there can also be multiple different interpretations of the same spectroscopic signal. The more of these types of ‘noise’ there is in the signal, the worse the signal-to-noise ratio (SNR) is. Noisy data—data with a low SNR—is a pronounced problem.
We’re still discovering different types of exoplanets and planetary atmospheres, and our models and analysis techniques aren’t complete. When combined with the low SNR problem, the pair comprise a major hurdle.
But machine learning can help, according to new research. “Machine-assisted classification of potential biosignatures in earth-like exoplanets using low signal-to-noise ratio transmission spectra” is a paper under review by the Monthly Notices of the Royal Astronomical Society. The lead author is David S. Duque-Castaño from the Computational Physics and Astrophysics Group at the Universidad de Antioquia in Medellin, Colombia.
The JWST is our most powerful transmission spectroscopy tool, and it’s delivered impressive results. But there’s a problem: observing time. Some observing efforts take an enormous amount of time. It can take a prohibitively high number of transits to detect things like ozone. If we had unlimited amounts of observing time, it wouldn’t matter so much.
One study showed that in the case of TRAPPIST-1e, it can take up to 200 transits to obtain statistically significant detections. The transit number becomes more reasonable if the search is restricted to methane and water vapour. “Studies have demonstrated that using a reasonable number of transits, the presence of these atmospheric species, which are typically associated with a global biosphere, can be retrieved,” the authors write. Unfortunately, methane isn’t as robust a biosignature as ozone.
Given the time required to detect some of these potential biomarkers, the researchers say that it might be better to use the JWST to conduct signal-to-noise ratio (SNR) surveys. “Although this may not allow for statistically significant retrievals, it would at least enable planning for future follow-up observations of interesting targets with current and future more powerful telescopes (e.g., ELT, LUVOIR, HabEx, Roman, ARIEL),” the authors write, invoking the names of telescopes that are in the building or planning stages.
The researchers have developed a machine-learning tool to help with this problem. They say it can fast-track the search for habitable worlds by leveraging the power of AI. “In this work, we developed and tested a machine-learning general methodology intended to classify transmission spectra with low Signal-to-Noise Ratio according to their potential to contain biosignatures,” they write.
Since much of our exoplanet atmosphere spectroscopy data is noise, the ML tool is designed to process it, figure out how noisy it is, and classify atmospheres that may contain methane, ozone, and/or water or as interesting enough for follow-up observations.
The team generated one million synthetic atmospheric spectra based on the well-known TRAPPIST-1 e planet and then trained their ML models on them. TRAPPIST-1e is similar in size to Earth and is a rocky planet in the habitable zone of its star. “The TRAPPIST-1 system has gained significant scientific attention
in recent years, especially in planetary sciences and astrobiology, owing to its exceptional features,” the paper states.
The TRAPPIST-1 star is known for hosting the highest number of rocky planets of any system we’ve discovered. For the researchers, it’s an ideal candidate for training and testing their ML models because astronomers can get favourable SNR readings in reasonable amounts of time. The TRAPPIST-1e planet is likely to have a compact atmosphere like Earth’s. The resulting models were successful and correctly identified transmission spectra with suitable SNR levels.
The researchers also tested their models on realistic synthetic atmospheric spectra of modern Earth. Their system successfully identified synthetic atmospheres that contained methane and/or ozone in ratios similar to those of the Proterozoic Earth. During the Proterozoic, the atmosphere underwent fundamental changes because of the Great Oxygenation Event (GOE).
The GOE changed everything. It allowed the ozone layer to form, created conditions for complex life to flourish and even led to the creation of vast iron ore deposits that we mine today. If other exoplanets developed photosynthetic life, their atmospheres should be similar to the Proterozoic Earth’s, so it’s a relevant marker for biological life. (The recent discovery of dark oxygen has serious implications for our understanding of oxygen as a biomarker in exoplanet atmospheres.)
In their paper, the authors describe the detection of oxygen or ozone as the ‘Crown Jewel’ of exoplanet spectroscopy signatures. But there are abiotic sources as well, and whether or not oxygen or ozone are biotic can depend on what else is in the signature. “To distinguish between biotic and abiotic O2, one can look for specific spectral fingerprints,” they write.
To evaluate the performance of their model, they need to know more than which exoplanet atmospheres are correctly identified (True) and which exoplanet atmospheres are falsely identified (False.)
The results also need to be categorized as either True Positives (TP) or True Negatives (TN), which are related to accuracy, or False Positives (FP) or False Negatives (FN), which are errors. To organize their data they created a classification system they call a Confusion Matrix.
“In the diagram, we introduce the category interesting to distinguish planets that deserve follow-up observations or in-depth analysis,” the authors explain. “We should recall again that is the focus of this work: we do not aim at detecting biosignatures using ML but at labelling planets that are interesting or not.”
One of the models was successful in identifying likely biosignatures in Proterozoic Earth spectra after only a single transit. Based on their testing, they explain that the JWST would successfully detect most “inhabited terrestrial planets observed with the JWST/NIRSpec PRISM around M-dwarfs located at distances similar or smaller than that of TRAPPIST-1 e.” If they exist, that is.
These results can refine the JWST’s future efforts. The researchers write that “machine-assisted strategies similar to those presented here could significantly optimize the usage of JWST resources for biosignature searching.” They can streamline the process and maximize the chances that follow-up observations can discover promising candidates. The telescope is already two years and seven months into its planned five-and-a-half-year primary mission. (Though the telescope could last for up to 20 years overall.) Anything that can optimize the space telescope’s precious observing time is a win.
All in all, the study presents a machine-learning model that can save time and resources. It quickly sifts through the atmospheric spectra of potentially habitable exoplanets. While it doesn’t identify which ones contain biomarkers, it can identify the best candidates for follow-up after only 1 to 5 transits, depending on the type of atmosphere. Some types would require more transits, but the model still saves time.
“Identifying a planet as interesting will only make the allocation of observing time of valuable resources such as JWST more efficient, which is an important goal in modern astronomy,” they write.
Written by Evan Gough/Universe Today.