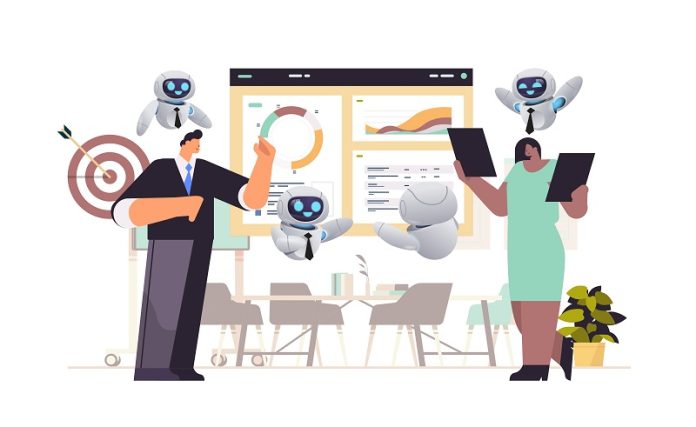
A research team has made a major breakthrough in semiconductor technology, developing analog hardware that can significantly enhance the performance of artificial intelligence (AI) while using less power.
This exciting advancement, which could have huge commercial potential, was detailed in a recent publication in Science Advances.
As AI technology rapidly advances, especially with applications like generative AI, the capabilities of current digital hardware like CPUs and GPUs are being stretched to their limits.
This has led researchers to explore analog hardware, which is specially designed for AI computations.
Analog hardware works by adjusting the resistance of semiconductors based on external voltage or current.
It uses a cross-point array structure with vertically crossed memory devices to process AI tasks simultaneously.
While this method offers benefits for certain computational tasks and continuous data processing, it has struggled to meet all the diverse needs of AI learning and inference.
To overcome these challenges, the research team, led by Professor Seyoung Kim from the Department of Materials Science and Engineering and the Department of Semiconductor Engineering, focused on a type of memory called Electrochemical Random Access Memory (ECRAM).
ECRAM controls electrical conductivity through the movement and concentration of ions, unlike traditional semiconductor memory.
ECRAM devices have a three-terminal structure with separate paths for reading and writing data, allowing them to operate at lower power levels.
The team successfully created ECRAM devices using this structure in a 64×64 array. Their experiments showed that the new hardware had excellent electrical and switching characteristics, high yield, and uniformity.
The team also applied a cutting-edge analog-based learning algorithm called the Tiki-Taka algorithm to this high-yield hardware. This approach maximized the accuracy of AI neural network training computations.
Additionally, they highlighted the “weight retention” property of their hardware, which ensures it doesn’t overload the artificial neural networks. This demonstrates the potential for commercializing this technology.
This research is particularly noteworthy because the largest ECRAM array reported before this was only 10×10. The team has now successfully implemented these devices on a much larger scale, showcasing varied characteristics for each device.
Professor Seyoung Kim of POSTECH remarked, “By developing large-scale arrays with new memory device technologies and creating analog-specific AI algorithms, we have identified the potential for AI computational performance and energy efficiency that far surpasses current digital methods.”
This development marks a significant step forward in the field of AI hardware, promising more efficient and powerful AI systems in the future.