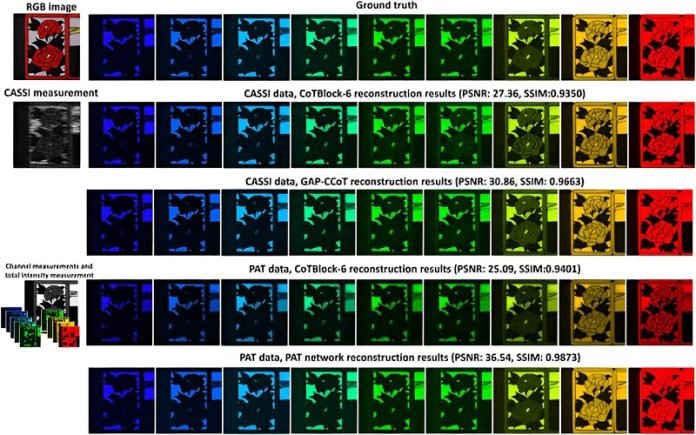
In the world of cameras, capturing detailed and accurate images can result in a massive amount of data.
This makes it tough to handle, store, and process these images.
To deal with this, researchers have always looked for ways to capture essential details without overwhelming the system.
One method to do this is called ‘sampling’, where specific bits of the image or scene are captured and later combined to recreate the whole picture. Think of it as putting together pieces of a puzzle.
A team of scientists, led by Minghao Hu from the University of Arizona and Zongliang Wu from Westlake University in Hangzhou, have discovered a smarter way to do this using neural networks.
Their findings are shared in the journal Intelligent Computing.
Traditional vs. New Methods
Traditionally, there were systems like CASSI and CACTI that specialized in capturing color or video details.
Imagine CASSI as using different colored glasses to see various colors in a scene. However, as technology evolves, these methods might soon become old-fashioned.
Enter neural networks, which are a bit like computerized brains. These networks can learn and make decisions, making them excellent for processing images.
The new system the researchers proposed uses a technique that’s simpler than the traditional methods. Instead of the “colored glasses” approach, they use something that’s more similar to taking pictures with two cameras that see slightly different things.
And the best part? These camera setups can be easily changed even while they’re in use.
To process the captured images, the team uses a special kind of neural network known as a “transformer” that’s designed for visual data. They’ve named their system ‘PAT’, which stands for Physics-Aware Transformer Network.
Testing the New Method
To see how good their new method was, the researchers compared it with the traditional ones.
For color pictures, they tested PAT against CASSI. The result? Both had their ups and downs. PAT was better at keeping the structure or shape of objects in the scene, while CASSI was better at maintaining the original colors and brightness.
For videos, PAT was set against CACTI. Interestingly, they both performed similarly. However, the big win for PAT was that it’s simpler and less expensive to use.
Moreover, the team showcased the power of PAT by recreating two different scenes, proving that it works well in real-world scenarios too.
Why Does This Matter?
When it comes to cameras, be it in our phones or professional setups, we always want better quality without slowing down the device or using too much storage.
This research shows a promising way to do that. By smartly capturing only essential parts and using computer brains (neural networks) to fill in the gaps, we can get high-quality images and videos without the usual challenges.
In simple words, the future of imaging might be cheaper, simpler, and just as good, if not better, thanks to neural networks like PAT.
Follow us on Twitter for more articles about this topic.