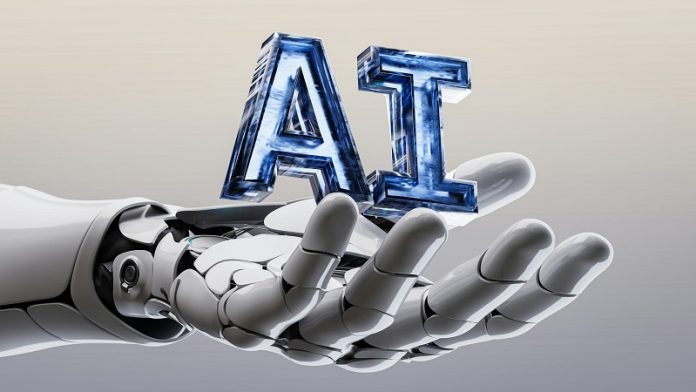
Can machines ever see the world like humans do?
New research suggests the answer may be yes.
A team of scientists from the University of Osaka has discovered that a type of artificial intelligence called a vision transformer (ViT) can learn to focus its visual attention in ways very similar to human gaze—without being given any examples or instructions.
When people look at an image or a scene, we don’t process everything at once. Instead, our eyes are drawn to important features like faces, bodies, or moving objects.
This skill is known as visual attention, and it helps us filter out unnecessary information to focus on what matters. While this comes naturally to humans, it’s a tough skill for AI to learn—especially without help.
But in a new study published in Neural Networks, the researchers showed that ViTs trained using a method called DINO—short for “self-distillation with no labels”—were able to learn this human-like visual attention all on their own.
DINO allows AI models to organize visual information by simply looking at large numbers of images, without needing any labels or human guidance.
To test how well the AI paid attention, the researchers compared its gaze patterns to those of 27 human adults who were shown short video clips.
The results were impressive. The ViTs trained with DINO focused on many of the same areas that humans did—like faces, outlines of people, and even background details—while traditional AI models trained with supervision did not.
Lead researcher Takuto Yamamoto explained that the DINO-trained AI developed specialized “attention heads” that naturally focused on different parts of the scene, much like our own visual systems. One group paid attention to faces, another to full human figures, and a third to background scenery. This kind of division closely mirrors how the human brain organizes visual information.
Even more remarkably, the AI learned to prioritize faces even though it was never told what a face is. According to senior author Shigeru Kitazawa, this happened because focusing on faces tends to provide useful information about the environment—just like it does for humans. This suggests that self-supervised learning might be tapping into the same basic strategies humans use to make sense of the world.
This research could lead to better, more human-aware AI systems. For example, robots that understand where people are looking might communicate more naturally, or educational tools could be designed to better support child development.
It also gives scientists a new way to study how human perception works—by watching how machines learn to see.