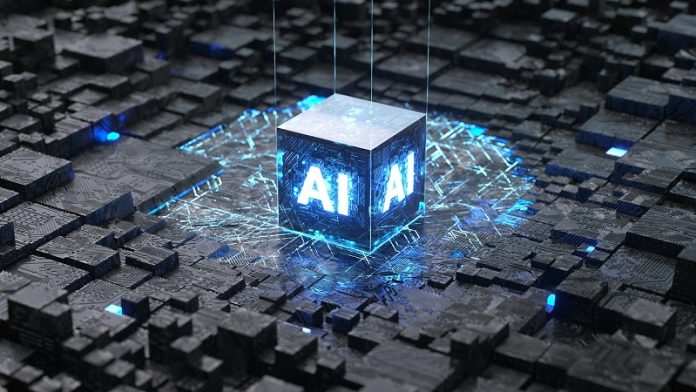
A new study has found a way to train large AI models, like the GPT series, using up to 30% less energy—without taking any extra time or reducing model accuracy.
This more efficient method could save enough energy to power 1.1 million U.S. homes by 2026, based on Wells Fargo’s projections of AI power demand.
This breakthrough could also help tackle climate change.
Data centers, which house the processors powering AI, could produce 1.2% of the world’s carbon emissions by 2027, according to the International Monetary Fund.
They also consume large amounts of water for cooling. Reducing energy waste in AI could lower both emissions and water use.
AI has the potential to help fight climate change by improving supply chains, managing energy use, and advancing climate research. However, these benefits don’t justify wasting energy. Some of the power used during AI training serves no real purpose and doesn’t affect the training time or the quality of the models.
“Why waste energy when it doesn’t help?” asks Mosharaf Chowdhury, a computer science professor at the University of Michigan and lead author of the study presented at the 30th Symposium on Operating Systems Principles. He warns that continuing to build larger data centers may not be sustainable. By using less energy for AI, we can reduce its carbon footprint, cut cooling needs, and make more efficient use of current energy resources.
The energy waste happens because training large AI models requires dividing tasks among many processors, often tens of thousands.
These processors, called GPUs, are specially designed for handling big data. But dividing tasks evenly among them is nearly impossible. Some processors may have light workloads, while others are overloaded, creating inefficiencies.
To solve this, the researchers created a software tool called Perseus. Perseus identifies the processors that are handling the most complex tasks, known as the “critical path,” and slows down processors with lighter loads. By synchronizing their completion times, Perseus eliminates unnecessary energy use.
“Reducing AI’s power cost also makes it more accessible,” Chowdhury explains. Smaller countries or organizations that can’t afford large power needs might rely on less advanced models. Efficient training tools like Perseus could help bridge this gap.
The researchers tested Perseus on several models, including GPT-3, and found it effective. Perseus is now available as part of a free tool called Zeus, designed to measure and optimize AI energy use.
This study was funded by organizations like the National Science Foundation and Mozilla Foundation and used resources from platforms like Chameleon Cloud and CloudLab.
By improving AI’s energy efficiency, Perseus could play a significant role in reducing its environmental impact while making advanced AI more widely accessible.
Source: University of Michigan.