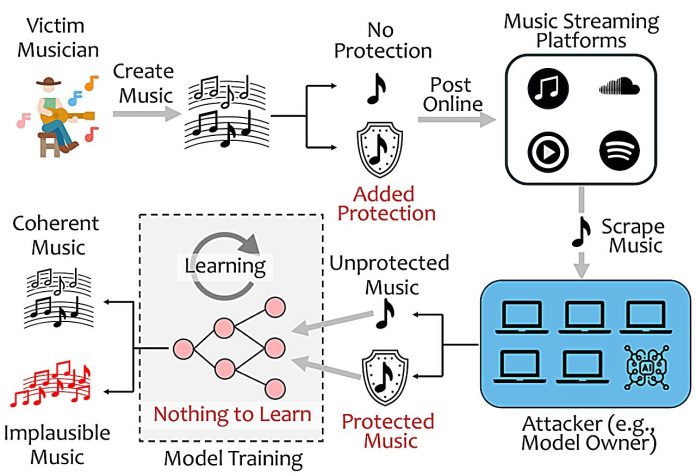
Generative AI has become so advanced that it recently completed Beethoven’s unfinished Tenth Symphony in a way that even music experts couldn’t distinguish between the AI-generated parts and Beethoven’s original notes.
This kind of AI uses massive libraries of data, including music, to learn and create new content. The problem is, much of the music available online is copyrighted, yet companies often train AI models using this protected content without proper permissions.
To address this issue, researchers have developed HarmonyCloak, a program that makes songs “unlearnable” by AI without affecting how they sound to human listeners.
This means the music can’t be copied or mimicked by AI models, while artists can still share their work with the public.
Generative AI models, like those used to create new songs or artworks, work by learning from large amounts of data.
This includes songs, which are often gathered from the internet. Companies might buy these songs legally, but the purchase only gives them a license for personal use—not the right to use the songs to train AI. However, this restriction is often ignored.
The result? AI-generated songs that sound a lot like the original human-created pieces, raising legal and ethical concerns for artists and their work. Although some states, like Tennessee, have begun to pass laws protecting vocal tracks from unauthorized AI use, entire songs still need protection.
Jian Liu, an assistant professor at the Min H. Kao Department of Electrical Engineering and Computer Science (EECS) at the University of Tennessee, worked with his Ph.D. student Syed Irfan Ali Meerza and Lichao Sun from Lehigh University to create HarmonyCloak.
This program tricks AI models into thinking there’s nothing new to learn from a song, even if the song is fed into the AI.
Liu explained that generative AI, like humans, can recognize when something is new information or something it already knows. AI models are designed to learn as much as possible from any new data. HarmonyCloak’s idea is to minimize this “knowledge gap” so that the AI thinks the song is already familiar and not worth learning.
One of the challenges was to develop a method that wouldn’t change the music’s sound for human listeners.
HarmonyCloak uses what are called “undetectable perturbations.” This involves adding very slight changes or new notes that are masked by the original song’s notes, making them hard for AI to detect.
Humans, on the other hand, are unable to hear extremely quiet sounds or sounds outside certain frequencies. HarmonyCloak uses this limitation to its advantage by introducing notes that blend seamlessly into the original music. According to Liu, “Our system preserves the quality of music because we only add imperceptible noises.”
To see how well HarmonyCloak worked, Liu and his team tested it with three advanced AI models and 31 human volunteers. They found that the human listeners rated the original and protected versions of the songs similarly for sound quality.
Meanwhile, the AI-generated music based on the “unlearnable” songs performed much worse, earning lower ratings from both human listeners and statistical metrics. This showed that HarmonyCloak successfully stopped the AI from learning the music while keeping the sound quality intact for human audiences.
Liu’s team believes HarmonyCloak is an ideal solution for protecting artists’ work in an age where AI is becoming more capable of generating convincing imitations. By making songs unlearnable to AI, artists can still share their music without worrying about unauthorized copying or AI mimicking their style.
“These findings underscore the substantial impact of unlearnable music on the quality and perception of AI-generated music,” Liu said. From a composer’s perspective, HarmonyCloak offers the best of both worlds: AI can’t learn from their songs, but listeners can still enjoy them.
Liu, Meerza, and Sun will present their research at the 46th IEEE Symposium on Security and Privacy (S&P) in May 2025. This tool could mark a major step forward in protecting artistic expression from unauthorized AI use.