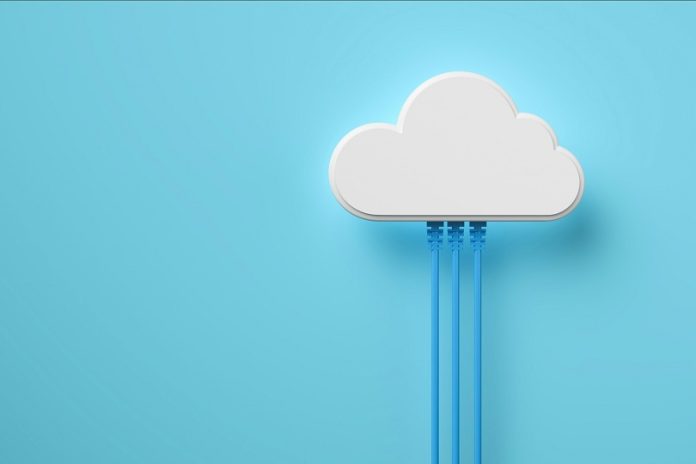
Researchers at MIT have developed a groundbreaking security protocol that could transform the way sensitive data is handled during cloud-based deep-learning computations.
This new system uses the quantum properties of light to ensure that data remains secure, making it particularly valuable for industries like healthcare, where privacy is a major concern.
Deep-learning models, which are used in everything from healthcare diagnostics to financial forecasting, require enormous computational power.
This often means relying on powerful cloud servers to process data.
However, this dependency on cloud computing brings significant security risks, especially when dealing with confidential information like patient data.
To address these risks, MIT researchers led by postdoctoral scholar Kfir Sulimany have created a protocol that uses the quantum nature of light to protect data during its journey to and from cloud servers.
By encoding data into laser light, which is commonly used in fiber optic communication systems, the protocol ensures that any attempt to copy or intercept the data will be detected, thanks to the fundamental principles of quantum mechanics.
One of the most impressive aspects of this new protocol is that it secures data without sacrificing the accuracy of deep-learning models.
In tests, the researchers found that their protocol could maintain 96% accuracy in predictions while still providing strong security.
“Deep learning models like GPT-4 have incredible capabilities but require massive computational resources,” said Sulimany.
“Our protocol allows users to harness these powerful models without compromising the privacy of their data or the proprietary nature of the models themselves.”
The research, recently presented at the Annual Conference on Quantum Cryptography, was conducted by Sulimany and a team of collaborators, including MIT postdoc Sri Krishna Vadlamani, former postdoc Ryan Hamerly (now at NTT Research, Inc.), MIT graduate student Prahlad Iyengar, and senior author Professor Dirk Englund.
The protocol was designed to secure data in a scenario where two parties are involved: a client who has confidential data (like medical images) and a central server that operates a deep-learning model.
The client wants to use the model to make a prediction, such as diagnosing cancer from medical images, without revealing the sensitive data to the server. At the same time, the server wants to protect its proprietary model from being copied.
The challenge with digital computation is that data can be easily copied or intercepted. However, quantum information cannot be perfectly cloned, a principle known as the no-cloning theorem. The MIT team leveraged this principle in their security protocol.
The protocol works by having the server encode the weights of a deep neural network (the core components that perform calculations) into an optical field using laser light.
This encoded information is sent to the client, who then uses it to make predictions based on their private data. Importantly, the client can only measure the light necessary to run the deep neural network, preventing them from copying the entire model.
The residual light, which doesn’t reveal any of the client’s data, is then sent back to the server for security checks.
In practical terms, the protocol is highly feasible because modern telecommunications equipment already uses optical fibers and lasers, so no special hardware is required.
The researchers found that their protocol could effectively secure data both ways—protecting the client’s data from the server and the server’s model from the client. The minimal leakage of information during the process is not enough for an attacker to recover any significant data.
Looking ahead, the MIT team is interested in exploring how this protocol could be applied to federated learning, where multiple parties contribute data to train a central deep-learning model. They are also considering how quantum operations, rather than classical ones, could further enhance both the accuracy and security of their system.
“This work cleverly combines techniques from deep learning and quantum key distribution to add a security layer while allowing for realistic implementation,” said Eleni Diamanti, a research director at Sorbonne University in Paris, who was not involved in the study.
Supported by the Israeli Council for Higher Education and the Zuckerman STEM Leadership Program, this research opens new possibilities for securing data in distributed AI systems, potentially transforming industries that rely on cloud-based computations.