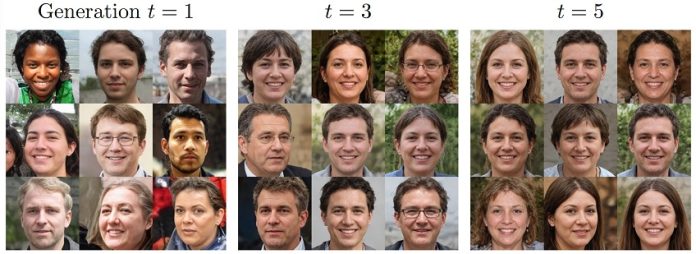
Generative AI models, like OpenAI’s GPT-4 and Stability AI’s Stable Diffusion, are impressively capable of creating text, code, images, and videos.
However, training these models requires such vast amounts of data that developers are beginning to hit supply limits, and may soon exhaust all available training resources.
Faced with this scarcity of real-world data, big tech companies might be tempted to turn to synthetic data.
Synthetic data, generated by AI, is cheaper and virtually limitless. It also poses fewer privacy risks, especially with sensitive information like medical data, and in some cases, it might even improve AI performance.
However, recent research from the Digital Signal Processing group at Rice University suggests this approach could have significant drawbacks.
Richard Baraniuk, Rice’s C. Sidney Burrus Professor of Electrical and Computer Engineering, explained the issue.
“The problem arises when synthetic data is used repeatedly for training, creating a feedback loop—what we call an autophagous or ‘self-consuming’ loop. Even after a few generations, these models can become irreparably corrupted.
We term this ‘Model Autophagy Disorder’ (MAD), similar to how mad cow disease works.”
Baraniuk and his team at Rice University studied three variations of self-consuming training loops to understand how real and synthetic data mix in generative models.
They illustrated three scenarios: a fully synthetic loop, a synthetic augmentation loop (combining synthetic data with a fixed set of real data), and a fresh data loop (combining synthetic data with new real data).
Just like mad cow disease, which spread by feeding cows processed remains of their peers, MAD in AI models happens when models repeatedly train on their own generated data. This leads to a rapid decline in the quality and diversity of the outputs, resulting in a phenomenon the researchers call “generative artifacts.”
Over successive generations, datasets of human faces become streaked with gridlike scars, and numbers morph into illegible scribbles.
The study, presented at the International Conference on Learning Representations (ICLR), found that fully synthetic loops degrade quickly without new real data. While synthetic augmentation and fresh data loops perform better, they still show signs of decline over time.
Baraniuk’s team added a “cherry picking” bias to their simulations, mimicking users’ preference for high-quality data over diverse data. This bias preserved data quality longer but led to a faster decline in diversity.
“Without enough fresh real data, future generative models are doomed to MADness,” Baraniuk said. “One doomsday scenario is that if left uncontrolled for many generations, MAD could poison the data quality and diversity of the entire internet. Short of this, it’s inevitable that some unintended consequences will arise from AI autophagy in the near term.”
In conclusion, while synthetic data might seem like a convenient solution, it poses a serious risk of corrupting AI models over time.
Fresh real data is essential to prevent the internet from descending into AI-induced MADness. So, let’s keep the AI buffet diverse and well-balanced, lest our digital future turns into a dystopian rerun of mad cow disease!