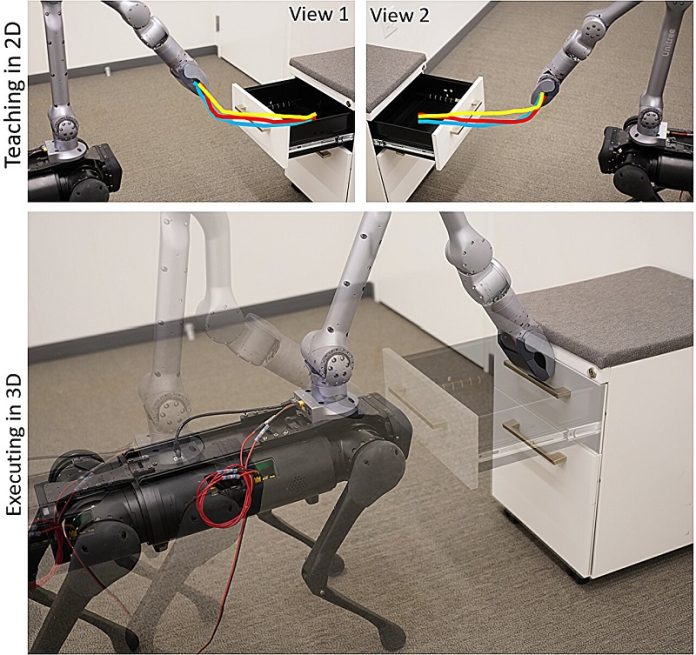
Researchers at Carnegie Mellon University’s Robotics Institute are revolutionizing the way robots learn to move by using a simple and intuitive method: sketching trajectories.
This new technique, which will be presented at the IEEE International Conference on Robotics and Automation in Yokohama, Japan, is making it easier to instruct robots without the need for complex programming or physical manipulation.
Traditionally, getting robots to move involves intricate programming that maps out paths they must follow to avoid obstacles and perform tasks.
Typically, this is done through kinesthetic teaching, where a person physically moves a robot’s joints into position, or teleoperation, which involves controlling the robot with a remote.
Both methods have limitations, especially when it comes to precision and ease of use.
The team at Carnegie Mellon, led by postdoctoral fellow William Zhi, Ph.D. student Tianyi Zhang, and RI Director Matthew Johnson-Roberson, proposes a simpler and more user-friendly approach.
They suggest teaching robots using sketches, a method humans often use to understand complex instructions through visual aids.
In their innovative approach, the researchers start by capturing images of the environment where the robot will operate.
Using two cameras placed at different angles, they take comprehensive snapshots. Then, they sketch the desired path of the robot directly on these images.
The sketches illustrate where the robot should go and what movements it should make, like opening a drawer or tipping over a box.
These sketches are then transformed into 3D models through a technique called ray tracing, which calculates distances and angles from the images. This conversion allows the robot to understand and follow the sketched paths in a three-dimensional space.
One practical application demonstrated by the researchers involved a quadruped robot—essentially a four-legged robot with an arm.
They taught this robot to perform various tasks like closing drawers and sketching letters, just by following the trajectories drawn on the images. Furthermore, the robot could adjust its gripper at the end of a trajectory to perform tasks like dropping objects into containers.
This method proved to be quite flexible and precise. The robot could start from different positions and still perform the tasks accurately, demonstrating the method’s adaptability to different scenarios and tasks.
This flexibility is crucial for practical applications in settings like manufacturing, where robots need to perform a variety of actions quickly and efficiently.
However, the technique currently works best with robots that have rigid joints. The researchers noted some challenges, such as the robot losing balance while performing certain tasks, which they plan to address in future improvements.
The potential of this method is significant, particularly in making robot programming accessible to people without specialized skills.
Imagine factory workers using an iPad to sketch instructions for robots, making it possible to collaborate easily and effectively. This could democratize the use of robotics in industries, making advanced robotics a tool that is accessible to a broader range of workers.
This research is not just about making robots perform better; it’s about simplifying the interface between humans and machines, paving the way for more interactive and flexible use of robotics in everyday tasks.