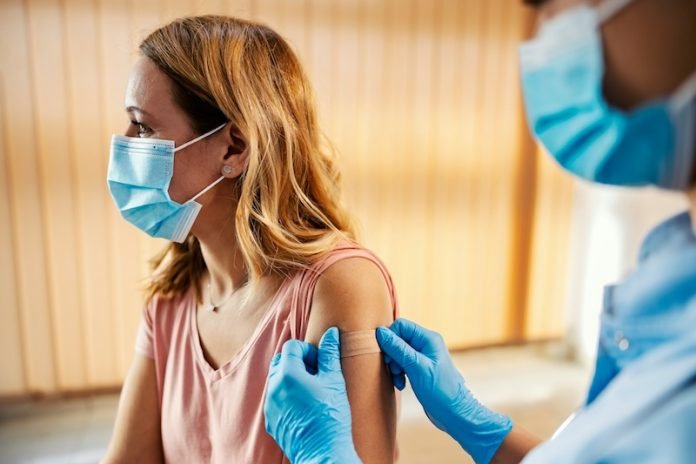
In a new step, scientists at Princeton University, led by machine learning expert Mengdi Wang, have taken artificial intelligence (AI) into a new realm: reading and optimizing the genetic code.
This innovation could revolutionize how we study biology and improve medical treatments, including the development of more effective vaccines against diseases like COVID-19.
The genetic code is the blueprint for life, containing instructions for creating all biological functions. It’s structured in a way that’s surprisingly similar to human languages, with its own grammar and syntax that dictate how sequences of DNA and RNA translate into proteins.
These proteins are the workhorses of cells, performing a vast array of functions necessary for life.
At the heart of this study is the use of AI, specifically language models, to analyze parts of the genome that don’t directly code for proteins but are crucial for controlling how efficiently those proteins are produced.
This area of study is particularly relevant for messenger RNA (mRNA) vaccines, which have been a key tool in the fight against COVID-19.
mRNA vaccines work by teaching our cells how to make a protein that triggers an immune response. However, not all of the mRNA sequence codes for the protein.
Some parts play a critical role in regulating how much protein is produced, and it’s these regions that the Princeton team targeted with their AI model.
After training their model on sequences from various species, the team was able to generate new mRNA sequences optimized for higher efficiency. Laboratory tests confirmed that these AI-designed sequences could indeed produce proteins more effectively, with some showing a 33% improvement over existing benchmarks.
This increase in efficiency could have significant implications for developing treatments for a wide range of diseases, not just COVID-19 but also other infectious diseases and cancers.
The success of this AI model highlights a broader potential: using AI to delve into the complexities of gene regulation. Gene regulation, the process by which certain genes are turned on or off, is fundamental to understanding disease and disorder.
The ability of AI to parse and optimize genetic sequences could open new avenues for research into the origins of various health conditions.
This project, which involved collaboration with researchers from the biotech firm RVAC Medicines and the Stanford University School of Medicine, represents a shift in how scientists approach the study of genetics.
Unlike conventional large language models trained on vast amounts of text from the internet, this model focused on a much narrower dataset: a few hundred thousand sequences related to the production of proteins.
Yet, it was able to uncover new insights into gene regulation and the efficiency of protein production.
The challenge, as described by Wang, was not just in analyzing the sequences themselves but in understanding the context and implications of different data types, such as efficiency measures and expression levels.
This required a novel approach to training the AI model, integrating diverse data sources into a coherent dataset for analysis.
The study, published in the journal Nature Machine Intelligence, marks a significant step forward in the intersection of AI, genetics, and medicine.
It demonstrates the power of AI to not just interpret complex biological information but to actively improve our understanding and manipulation of life’s fundamental codes. As this research progresses, it could lead to breakthroughs in how we diagnose, treat, and ultimately prevent a wide array of diseases.
The research findings can be found in Nature Machine Intelligence.
Copyright © 2024 Knowridge Science Report. All rights reserved.