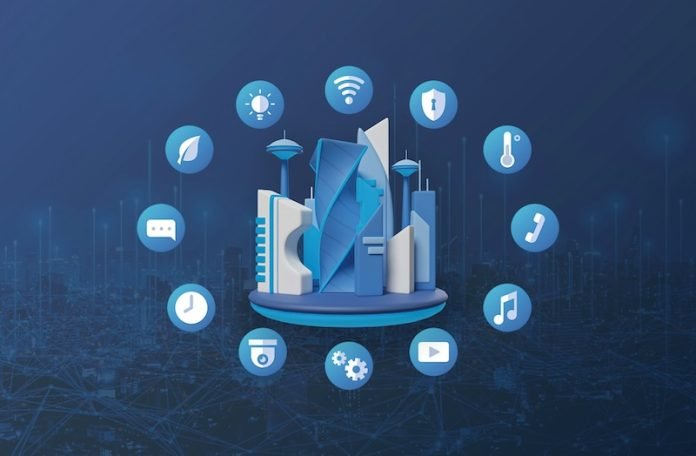
The widespread use of machine learning (ML) algorithms to predict potential connections within networks—whether among users on social media platforms, genes and proteins in biological research, or other types of interconnected systems—highlights the importance of accurate performance metrics.
However, recent findings from UC Santa Cruz’s Professor C. “Sesh” Seshadhri and Nicolas Menand challenge the reliability of the commonly used Area Under Curve (AUC) metric in evaluating the efficacy of link prediction tasks.
Link prediction operates by analyzing the existing connections within a network and employing ML algorithms to forecast future associations.
This task is not only central to the growth strategies of social media networks but also serves as a crucial benchmark in the scientific validation of new ML algorithms.
The efficiency of these algorithms is represented through low-dimensional vector embeddings, where entities within a network are mathematically mapped as vectors in a defined space, allowing for the manipulation and analysis essential to ML processes.
The AUC metric, which scores algorithm performance on a scale from zero to one, has been a standard tool for measuring the success of these predictions.
However, Seshadhri and Menand’s research, published in the Proceedings of the National Academy of Sciences, reveals significant mathematical limitations inherent in using low-dimensional embeddings for link predictions that AUC fails to account for.
This oversight suggests that AUC might overestimate the performance of link prediction tasks, presenting a misleadingly optimistic view of their effectiveness.
The authors argue that the mathematical constraints they’ve identified undermine the trustworthiness of decisions made based on AUC-measured link prediction performance.
They advocate for the abandonment of AUC in favor of a new, more comprehensive metric that can accurately reflect the capabilities and limitations of link prediction algorithms.
This call for a methodological shift has far-reaching implications for the field of ML, particularly for applications relying on network analysis and link prediction.
The introduction of a more accurate performance metric would not only enhance the reliability of link prediction tasks but also improve the overall trustworthiness of decision-making processes in ML applications.
As the field continues to evolve, the adoption of such metrics will be crucial in ensuring that the development and application of ML algorithms are both scientifically rigorous and practically effective.
The research findings can be found in PNAS.
Copyright © 2024 Knowridge Science Report. All rights reserved.