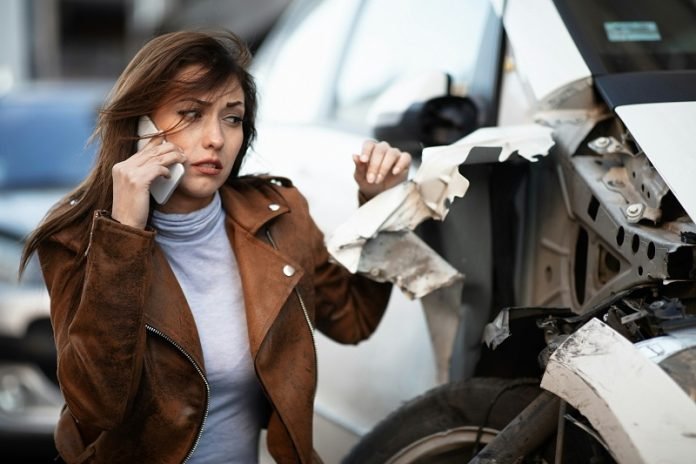
In a groundbreaking study by a team of engineers from the University of Massachusetts Amherst and Greek civil engineers, a new light is shed on what makes some roads more prone to accidents than others.
By harnessing the power of machine learning, they’ve pinpointed key factors that can predict where crashes are likely to happen.
This research isn’t just a technical achievement; it’s a potential lifesaver.
Imagine driving on a road where the speed limit suddenly drops without warning or trying to navigate lanes with faded markings.
These aren’t just minor inconveniences; according to the study, they’re significant predictors of where accidents are likely to occur.
The team’s analysis revealed that abrupt speed limit changes, damaged guardrails, and the dreaded “alligator” cracking on the pavement, along with unclear road signs and markings, are major red flags for road safety.
This wasn’t a small project.
The engineers examined an impressive dataset covering 9,300 miles of road across 7,000 locations in Greece, provided by Egnatia Odos, a major engineering firm. The depth of real-world data available for this study is rare and provided a unique opportunity to understand road safety on a large scale.
Assistant Professor Jimi Oke, one of the UMass Amherst researchers, believes these findings aren’t just relevant to Greece.
Given the universal nature of the road features examined, the insights could apply worldwide, including in the United States. This opens up exciting possibilities for improving road safety in various contexts and locations.
But the study does more than just highlight problems. It offers a blueprint for the future. By identifying the most critical road features linked to accidents, it directs where investment and attention should go to enhance road safety effectively.
This targeted approach means that funds can be used more efficiently, focusing on the issues that will make the most significant difference in reducing crash risks.
Looking ahead, the implications of this work are vast. The same machine learning techniques could be used to train artificial intelligence systems for real-time monitoring of road conditions, offering a proactive approach to identifying and fixing potential hazards before they result in accidents.
Associate Professor Simos Gerasimidis sees this as a prime example of AI’s potential to address real-world challenges. The study not only showcases what’s possible with AI in engineering but also acts as a call to action for officials to use these insights to make roads safer.
This collaborative effort between academia and engineering could pave the way for similar partnerships in the future, tackling societal issues with a combination of mathematical tools and real-world data.
It’s a powerful reminder of how technology, when applied thoughtfully, can make a significant impact on our lives, starting with making the roads we travel on every day safer for everyone.
Source: University of Massachusetts Amherst.