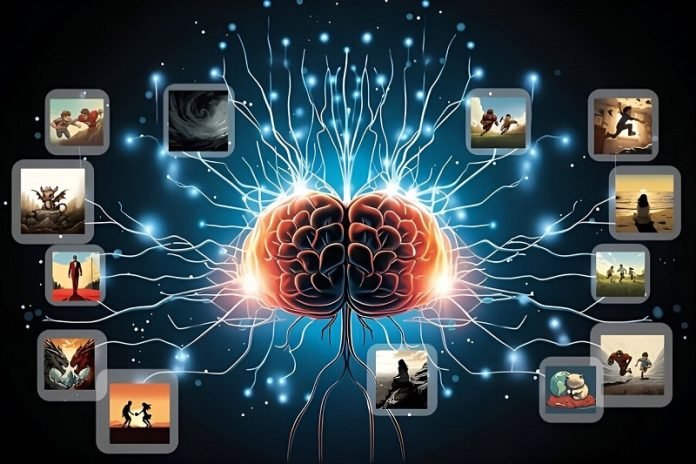
In a groundbreaking study by MIT researchers, a new method of training artificial intelligence (AI) models using synthetic images has outperformed the traditional approach of using real photographs.
This innovative approach, developed by a team including MIT Ph.D. student Lijie Fan, could revolutionize how AI models learn and understand the world.
The core of this new method is a system named StableRep.
It doesn’t just use any synthetic images; it generates them through advanced text-to-image models like Stable Diffusion. This process is akin to creating virtual worlds from written descriptions.
The secret ingredient of StableRep is a unique strategy called “multi-positive contrastive learning.”
Instead of simply feeding the model data, it helps the AI to understand high-level concepts by looking at the context and variations in the images.
The system views multiple images created from the same text as positive pairs. This means that the AI doesn’t just look at the pixels but dives deeper into understanding the concepts behind the images.
This method offers a significant advantage over traditional models, like SimCLR and CLIP, which have been trained on real images. In extensive testing, StableRep showed better results than these top-tier models.
The advantage of using synthetic images for AI training is enormous. In the past, researchers had to go through laborious processes to gather data, from manually capturing photographs to scouring the internet.
This method was not only time-consuming but often led to datasets with inaccuracies and societal biases. With StableRep, creating the necessary data for training AI models becomes as easy as typing a text command.
An important aspect of StableRep’s success lies in adjusting the “guidance scale” of the generative model. This fine-tuning ensures a balance between diversity and accuracy in the synthetic images. When done correctly, these images can be as effective or even more so than real images for training AI models.
The team took the method a step further by adding language supervision, creating an enhanced version called StableRep+. When trained with 20 million synthetic images, StableRep+ not only achieved higher accuracy but also proved to be more efficient compared to CLIP models trained with 50 million real images.
However, the journey isn’t without challenges. The team acknowledges issues like the slow pace of image generation, potential mismatches between text prompts and images, the risk of amplifying biases, and complexities in attributing images. These are all areas that need attention for future progress.
Despite these challenges, the approach holds promise. Starting with a good generative model, the team can repurpose it for new tasks, like training recognition models and visual representations. This reduces the initial dependence on large-scale real data.
Yet, the process is not free from biases. The choice of text prompts in the image synthesis process can influence the resulting images. This indicates the need for careful text selection or possibly human curation to minimize bias.
Using the latest text-to-image models, the researchers have achieved a new level of control over image generation. This method surpasses traditional image collection in efficiency and versatility.
It’s particularly useful for specialized tasks, such as balancing image variety in long-tail recognition, and presents a practical supplement to using real images for training.
This research signifies a significant step forward in visual learning. It highlights the potential for cost-effective training alternatives while underscoring the importance of improving data quality and synthesis.
David Fleet, a researcher at Google DeepMind and a professor at the University of Toronto, who was not involved in the study, reflects on the impact of this research.
He notes that this work provides compelling evidence that contrastive learning from massive amounts of synthetic image data can produce superior representations than those learned from real data.
This advancement opens up possibilities for improving a wide range of vision tasks and brings us closer to the dream of generative model learning.
Source: MIT.