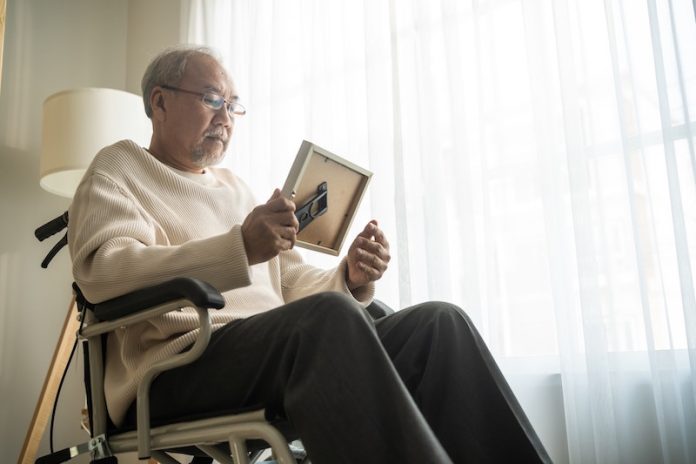
Older adults contending with late-life depression are thrust into a hazardous battle with suicidal thoughts, plans, and actions, making them a demographic with a notably high susceptibility to death by suicide.
As the shadow of depression extends over an increasing number of elders, an urgent call is sounded for efficacious and objective methodologies to screen suicide risk.
Traditionally, suicide risk screening has leaned heavily on structured clinical interviews and questionnaires – mechanisms that, while beneficial, are inherently time and effort-intensive.
Harnessing the Power of Brain Patterns in Suicide Risk Prediction
Enter Professor Tatia Lee and her team at the University of Hong Kong, whose groundbreaking research uncovers a promising horizon: brain patterns as predictive tools for suicide risk among those with late-life depression.
Published in Nature Mental Health, the study casts light on the potential of using whole-brain resting-state functional connectivity and white matter structural connectivity as reliable indicators for suicide risk prediction.
Both these techniques, underpinned by examining correlated brain signals and physical interconnections between brain regions respectively, have demonstrated a consistent performance in reliability and generalizability.
Involving a cohort of 91 patients diagnosed with major depressive disorder, and categorized as non-suicidal, suicidal ideation/plan, and those who have attempted suicide, the research employed brain connectivity profiles, subjected to machine learning, to classify the participants.
Remarkably, these profiles not only improved classification-prediction accuracy compared to the solitary use of questionnaires but also upheld their predictive validity in two independent datasets.
Beyond Conventional Screening: An Advanced, Multimodal Approach
For Professor Lee, time is of the essence in mitigating the fatal consequences of depression. As she notes, early identification of risk paves the way for timely, life-preserving interventions.
Therefore, the integration of functional and structural connectivity in classification not only sharpens accuracy but also encapsulates individual variances in suicide risk among late-life depression patients.
The future beckons with the prospects of more meticulous screening methods.
Envisaged is a paradigm where multimodal brain imaging, interlaced with psychological and behavioral data, coalesces into predictive models, which, in turn, yield precision suicide risk scores.
Looking Ahead: Personalized Assessment and Interventions
The path forward, illuminated by these innovative findings, involves propelling these predictive models through further testing and potential refinement. The ultimate goal?
To weave them into the fabric of clinical assessments, spawning a framework where individualized suicide risk scores navigate the creation of bespoke assessment and intervention programs.
As science inches closer to integrating these insights into practical applications, the possibility of identifying and saving individuals from the precipice of suicide becomes ever more tangible.
This research doesn’t just offer a new lens through which to understand and predict suicide risk – it provides hope, signaling a future where preemptive intervention could shield countless individuals from the darkest depths of depression.
If you care about depression, please read studies about how dairy foods may influence depression risk, and B vitamins could help prevent depression and anxiety.
For more information about mental health, please see recent studies that ultra-processed foods may make you feel depressed, and extra-virgin olive oil could reduce depression symptoms.
The research findings can be found in Nature Mental Health.
Follow us on Twitter for more articles about this topic.
Copyright © 2023 Knowridge Science Report. All rights reserved.