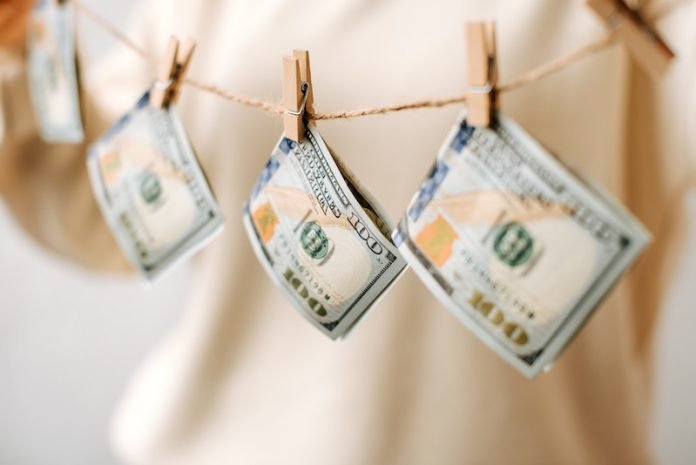
Computer scientists have pioneered a groundbreaking tool that can detect money laundering with unprecedented speed and accuracy, scanning 50 million transactions in under a second.
Developed by the Department of Informatics, this tool introduces an innovative approach, utilizing algorithms to swiftly spot instances of “smurfing,” a technique where large sums of money are fragmented into multiple smaller transactions across various bank accounts to evade detection.
Published in the Proceedings of the 2023 SIAM International Conference on Data Mining, the study, led by Dr. Huiping Chen and Dr. Grigorios Loukides, reveals that the software outperforms current detection methods by over three times and can process substantially larger datasets.
The tool focuses on suspicious activities within complex transaction graphs, tracking funds even when scattered across different accounts.
Existing anti-money laundering methods, predominantly rule-based or reliant on machine learning, often fall short, especially against novel or evolving tactics, due to their dependency on historical data and pre-set detection parameters.
The newly developed tool mitigates such limitations by providing an optimal, automated, and highly effective solution, enabling experts to survey vast amounts of data swiftly and identify malicious actors efficiently.
The software is open-source and has demonstrated its efficacy through tests on real and hypothetical data, detecting all suspicious transaction patterns.
Beyond financial security, researchers envision its applicability in optimizing retail marketing campaigns by analyzing and identifying the most lucrative product bundles.
Highlights
Innovative Tool: Researchers have developed a new anti-money laundering tool, capable of analyzing 50 million transactions in less than a second, based on algorithms designed to detect money “smurfing.”
Enhanced Detection: The software can trace funds accurately across multiple accounts and is over three times more effective than existing detection methods, capable of analyzing larger datasets.
Application and Testing: The tool, tested on real and hypothetical data, has proven effective in detecting suspicious transaction patterns and has potential applications in optimizing retail strategies by identifying lucrative product combinations.
Open Source: The software is freely accessible and can be applied to extensive datasets, offering more rapid and precise analysis compared to conventional methods.
Detailed Insight
This development represents a significant stride in combatting money laundering, a global challenge with the United Nations Office on Drugs and Crime (UNODC) estimating that between 2% and 5% of global GDP is laundered annually, equivalent to £632 billion to over £1.5 trillion.
The tool not only offers a quicker and more reliable way of detecting illicit financial activities but also provides a heightened sense of security to financial institutions, allowing them to identify and counteract malicious financial activities more efficiently.
Dr. Loukides emphasizes that the tool is far more automated and enables much faster analysis of data compared to existing options.
This acceleration in the analytical process empowers money laundering experts to identify actors with malicious intent effectively.
By further refining this technology, the research team aims to deliver a solution with even higher accuracy and speed than current methodologies.
Beyond its primary application in detecting financial irregularities, the innovative tool also holds promise in enhancing retail strategies by identifying the most profitable bundles of products, demonstrating its multifaceted applicability and potential impact across various domains.
The research findings can be found in the Proceedings of the 2023 SIAM International Conference on Data Mining.