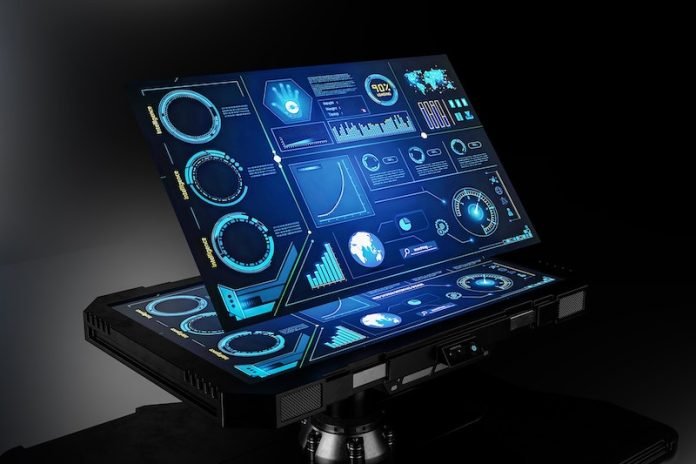
Scientists from UCLA and the United States Army Research Laboratory have outlined a novel approach to improve AI-powered computer vision.
The new method involves incorporating physics-based awareness into data-driven techniques.
The research, published in Nature Machine Intelligence, could significantly improve how AI machinery senses, interacts, and responds to its environment in real-time.
Issues with Traditional AI Techniques
Traditional computer vision techniques allow AIs to make sense of their surroundings by decoding data and inferring properties of the physical world from images.
Although images are formed through the physics of light and mechanics, most computer vision techniques have focused primarily on data-based machine learning.
Meanwhile, separate physics-based research has explored the various physical principles behind many computer vision challenges.
Incorporating an understanding of physics into neural networks has remained a challenge.
New Hybrid Approach
The UCLA study proposes a hybrid methodology, leveraging both deep knowledges from data and real-world physics insights to create an AI with enhanced capabilities.
The team outlined three ways to integrate physics and data into computer vision AI:
- Tagging objects in AI data set with additional physics-related information, such as their speed or weight.
- Running data through a network filter that codes physical properties into what cameras capture.
- Using physics-based knowledge to help AI interpret training data on what it observes.
Potential Impact
The study’s corresponding author Achuta Kadambi, an assistant professor of electrical and computer engineering at the UCLA Samueli School of Engineering, noted that “Physics-aware forms of inference can enable cars to drive more safely or surgical robots to be more precise.”
With this hybrid approach, AI could track and predict an object’s motion more accurately and produce high-resolution images from scenes obscured by adverse weather conditions.
In the long term, the researchers suggest that AI could even start learning the laws of physics independently.
Other authors on the paper include Army Research Laboratory computer scientist Celso de Melo, and UCLA faculty members Stefano Soatto, Cho-Jui Hsieh, and Mani Srivastava.
The study was published in Nature Machine Intelligence.
Copyright © 2023 Knowridge Science Report. All rights reserved.