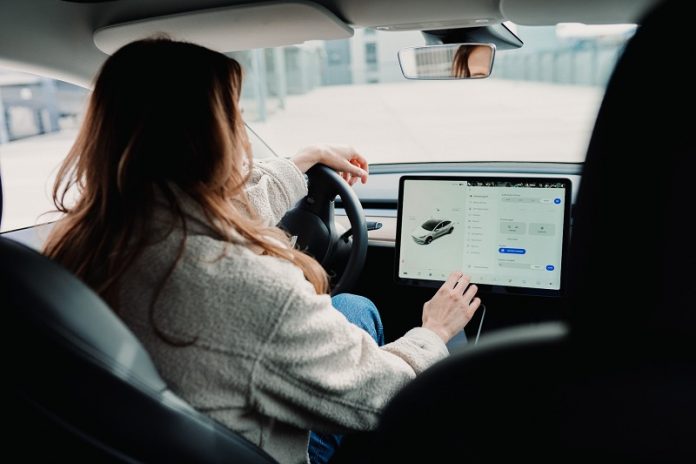
The rapid growth of mobile technologies has led to the generation of massive amounts of consumer location data.
This data is valuable for businesses and advertisers but raises concerns about individuals’ privacy.
In response, researchers from Carnegie Mellon University, the University of Virginia, and New York University have developed a machine learning framework to quantify privacy risks, preserve data utility, and protect consumers.
Their innovative approach significantly reduces privacy risks while maintaining the usefulness of data for advertisers.
This study offers a crucial tool to ensure a secure and privacy-aware environment in the multi-billion-dollar location data ecosystem.
The value and risks of location data
The use of smartphones and location-based services generates extensive mobile location data, enabling applications such as personalized recommendations and location-based advertising.
However, sharing location data can expose individuals’ personally identifiable information, leading to privacy risks. Malicious actors may exploit this data for short-term gains, necessitating a framework that balances risks and utilities for different stakeholders involved.
A personalized and flexible framework
The researchers created a machine learning-based framework that assesses individuals’ privacy risks and quantifies advertisers’ utility.
The framework incorporates a personalized obfuscation scheme that suppresses certain visited locations based on an individual’s risk level. It also accommodates different types of risks and utilities while respecting acceptable levels set by consumers and advertisers.
Testing and validation
To evaluate the framework, the researchers partnered with a leading data aggregator that integrates location data from various mobile apps.
The dataset, collected over five weeks, covered a significant metropolitan area and represented a quarter of the U.S. population.
By analyzing a million trajectories of 40,000 consumers, the researchers demonstrated that their framework outperformed previous methods proposed in recent studies.
Protecting privacy and preserving utility
Using the proposed framework, data aggregators can effectively protect consumer privacy by obfuscating data while ensuring its utility for advertisers.
The framework allows for personalized and diverse demands from both consumers and advertisers, accommodating various types of risks, utilities, and tradeoffs.
It offers a crucial tool for privacy-aware big data location-based applications and services, striking a balance between privacy concerns and data usefulness.
Although the study provides valuable insights, the authors acknowledge its limitations.
The dataset used did not include demographic information, which could provide a deeper understanding of privacy issues.
Furthermore, the framework focused on one-time data sharing with advertisers and did not account for complex scenarios involving multiple risks, utilities, or combined data from different sources.
As location-based marketing becomes increasingly prevalent, it is essential to address the privacy concerns associated with location data.
The researchers’ framework offers a groundbreaking solution to protect privacy and preserve data utility.
By using advanced machine learning techniques, this framework ensures a secure environment for location-based applications and services, benefiting both consumers and advertisers.