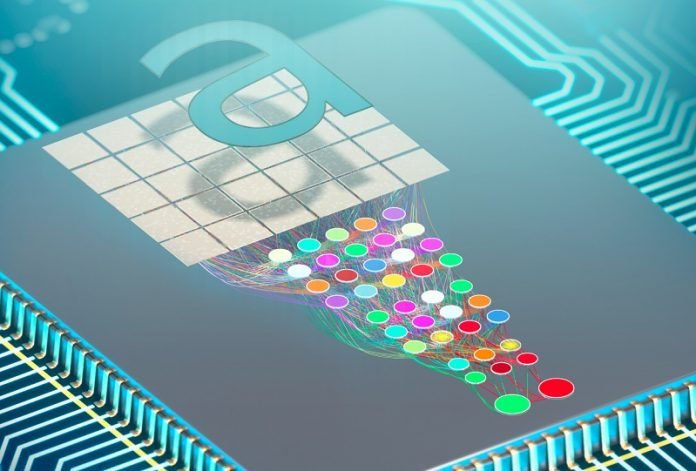
Artificial intelligence (AI) plays an important role in many systems, from predictive text to medical diagnoses.
Inspired by the human brain, many AI systems are implemented based on artificial neural networks, where electrical equivalents of biological neurons are interconnected, trained with a set of known data, such as images, and then used to recognize or classify new data points.
In traditional neural networks used for image recognition, the image of the target object is first formed on an image sensor, such as the digital camera in a smart phone.
Then, the image sensor converts light into electrical signals, and ultimately into the binary data, which can then be processed, analyzed, stored, and classified using computer chips.
Speeding up these abilities is key to improving any number of applications, such as face recognition, automatically detecting text in photos, or helping self-driving cars recognize obstacles.
While current, consumer-grade image classification technology on a digital chip can perform billions of computations per second, making it fast enough for most applications, more sophisticated image classification such as identifying moving objects, 3D object identification, or classification of microscopic cells in the body, are pushing the computational limits of even the most powerful technology.
The current speed limit of these technologies is set by the clock-based schedule of computation steps in a computer processor, where computations occur one after another on a linear schedule.
To address this limitation, Penn Engineers have created the first scalable chip that classifies and recognizes images almost instantaneously.
Firooz Aflatouni, associate professor in electrical and systems engineering, along with postdoctoral fellow Farshid Ashtiani and graduate student Alexander J. Geers, have removed the four main time-consuming culprits in the traditional computer chip: the conversion of optical to electrical signals, the need for converting the input data to binary format, a large memory module, and clock-based computations.
They have achieved this through direct processing of light received from the object of interest using an optical deep neural network implemented on a 9.3 square millimeter chip.
The study, published in Nature, describes how the chip’s many optical neurons are interconnected using optical wires or “waveguides” to form a deep network of many “neuron layers” mimicking that of the human brain. Information passes through the layers of the network, with each step helping to classify the input image into one of its learned categories.
In the researchers’ study, the images the chip classified were of hand-drawn, letter-like characters.
Written by Melissa Pappas.