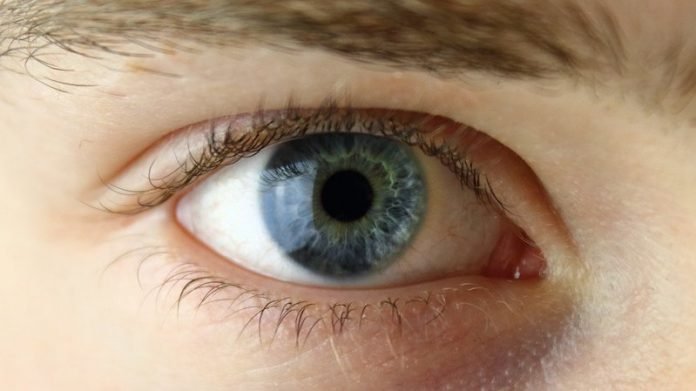
In a new study, researchers created a novel deep learning method that makes automated screenings for eye diseases such as diabetic retinopathy more efficient.
Reducing the amount of expensive annotated image data that is required for the training of the algorithm, the method is attractive for clinics.
In the use case of diabetic retinopathy, the researchers developed a screening algorithm that needs 75% less annotated data and achieves the same diagnostic performance of human experts.
The research was conducted by a team at the Technical University of Munich and elsewhere.
Diabetic retinopathy is a diabetes-related eye disease damaging the retina and can ultimately lead to blindness.
Measuring the retinal thickness is an important procedure to diagnose the disease in risk patients. To do so, most clinics take photographs of the fundus—the surface of the back of the eye.
In order to automate the screening of these images, clinics started to apply deep learning algorithms.
These algorithms require large sets of fundus images with expensive annotations in order to be trained to screen correctly.
In the study, the team used a population-size data set containing over 120,000 unannotated fundus and co-registered OCT images.
OCT (optical coherence tomography) allows for precise information about the retinal thickness but is not commonly available in every eye care center.
The researchers developed a novel method called “cross-modal self-supervised retinal thickness prediction” and applied it to pre-train a deep learning algorithm with the data set.
In this use case, cross-modal self-supervised learning allowed the algorithm to teach itself to recognize unannotated fundus images with different OCT-derived retinal thickness profiles, predicting the thickness information directly from the fundus.
By accurately predicting retinal thickness, a key diagnostic feature for diabetic retinopathy, the algorithm was then able to learn how to predict screening outcomes.
This novel method shrunk the need for expensive annotated data to train the deep learning algorithm by 75%.
When applied in automated screenings for diabetes retinopathy, it achieved the same diagnostic performance, both, compared to previous algorithms which had required much more training data and compared to human experts.
The team says the use case in diabetic retinopathy is ready for immediate use in clinics and is a perfect example of how AI can improve the daily business of clinics and thus everybody’s health.
Beyond diabetic retinopathy, the novel method allows for further clinical applications where much-unannotated data is available but expert annotations are scarce, such as age-related macular degeneration (AMD).
One author of the study is Olle Holmberg.
The study is published in Nature Machine Intelligence.
Copyright © 2020 Knowridge Science Report. All rights reserved.