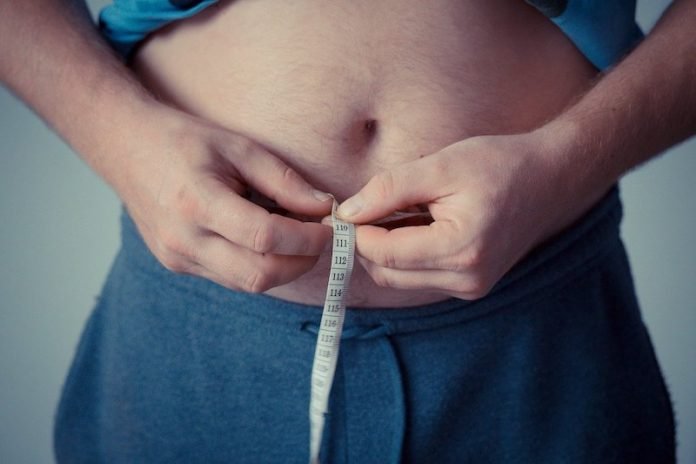
In a new study, researchers found that AI belly fat measures could predict heart attack and stroke effectively.
They found an automated deep learning analysis of abdominal CT images produces a more precise measurement of body composition and predicts heart attack and stroke, better than overall weight or body mass index (BMI).
The research was conducted by a team at the University of California San Francisco.
Unlike BMI, which is based on height and weight, a single axial CT slice of the abdomen visualizes the volume of the subcutaneous fat area, visceral fat area, and skeletal muscle area.
However, manually measuring these individual areas is time-intensive and costly.
In the study, the team developed a fully automated method using deep learning—a type of artificial intelligence (AI)—to determine body composition metrics from abdominal CT images.
Abdominal CT scans that are routinely performed provide a more granular way of looking at body composition.
The data were derived from the 33,182 abdominal CT outpatient exams performed on 23,136 patients in 2012.
The researchers identified 12,128 patients who were free of major heart diseases and cancer diagnoses at the time of imaging.
Patients were then divided into four quartiles based on the normalized values of the subcutaneous fat area, visceral fat area, and skeletal muscle area.
In the study, it was determined which of these 12,128 patients had a heart attack or stroke within 5 years after their index abdominal CT scan.
The researchers found 1,560 heart attacks and 938 strokes occurred in this study group.
Statistical analysis demonstrated that the visceral fat area was linked to future heart attack and stroke. BMI was not linked to a heart attack or stroke.
The group of patients with the highest proportion of visceral fat area were more likely to have a heart attack.
The group of patients with the lowest amount of visceral fat area were protected against stroke in the years following the abdominal CT exam.
These results show that precise measures of body muscle and fat compartments achieved through CT outperform traditional biomarkers for predicting risk for heart disease and stroke.
According to the team, this work demonstrates that fully automated and normalized body composition analysis could now be applied to large-scale research projects.
One author of the study is Kirti Magudia, M.D., Ph.D., an abdominal imaging, and ultrasound fellow.
The study was presented at the annual meeting of the Radiological Society of North America (RSNA).
Copyright © 2020 Knowridge Science Report. All rights reserved.