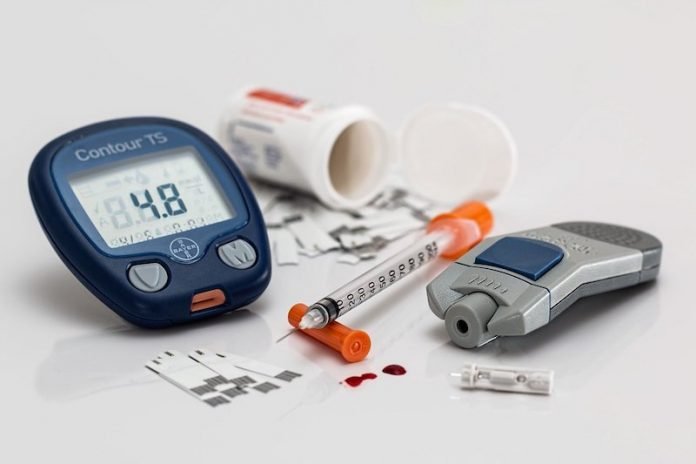
In a new study, researchers have developed a ‘digital biomarker’ that would use a smartphone’s built-in camera to detect Type 2 diabetes.
It potentially provides a low-cost, in-home alternative to blood draws and clinic-based screening tools.
The research was conducted by a team at UC San Francisco.
Type 2 diabetes affects more than 32 million Americans and more than 450 million people worldwide and can raise the risk of diseases affecting nearly every organ system, including coronary heart disease, kidney failure, blindness, and stroke.
In the current pandemic, it also has been found to increase the risk of severe symptoms of COVID-19. Yet, half of the people with diabetes are unaware of their diagnosis and risks to their health.
Screening tools that can be deployed easily, using technology already contained in smartphones, could rapidly increase the ability to detect diabetes, including populations out of reach of traditional medical care.
In developing the biomarker, the researchers hypothesized that a smartphone camera could be used to detect vascular damage due to diabetes by measuring signals called photoplethysmography (PPG), which most mobile devices, including smartwatches and fitness trackers, are capable of acquiring.
The researchers used the phone flashlight and camera to measure PPGs by capturing color changes in the fingertip corresponding with each heartbeat.
In the study, they obtained nearly 3 million PPG recordings from 53,870 patients in the Health eHeart Study who used the Azumio Instant Heart Rate app on the iPhone and reported having been diagnosed with diabetes by a health care provider.
This data was used to both develop and validate a deep-learning algorithm to detect the presence of diabetes using smartphone-measured PPG signals.
Overall, the algorithm correctly identified the presence of diabetes in up to 81% of patients in two separate datasets.
When the algorithm was tested in an additional dataset of patients enrolled from in-person clinics, it correctly identified 82% of patients with diabetes.
Among the patients that the algorithm predicted did not have diabetes, 92% to 97% indeed did not have the disease across the validation datasets.
When this PPG-derived prediction was combined with other easily obtainable patient information, such as age, gender, body mass index and race/ethnicity, predictive performance improved further.
At this level of predictive performance, the team said the algorithm could serve a similar role to other widespread disease screening tools to reach a much broader group of people, followed by a physician’s confirmation of the diabetes diagnosis and a treatment plan.
They recommend further study to determine the effectiveness of this approach for specific clinical applications, such as screening or therapeutic monitoring.
One author of the study is Geoffrey H. Tison, MD, MPH, an assistant professor in cardiology.
The study is published in Nature Medicine.
Copyright © 2020 Knowridge Science Report. All rights reserved.