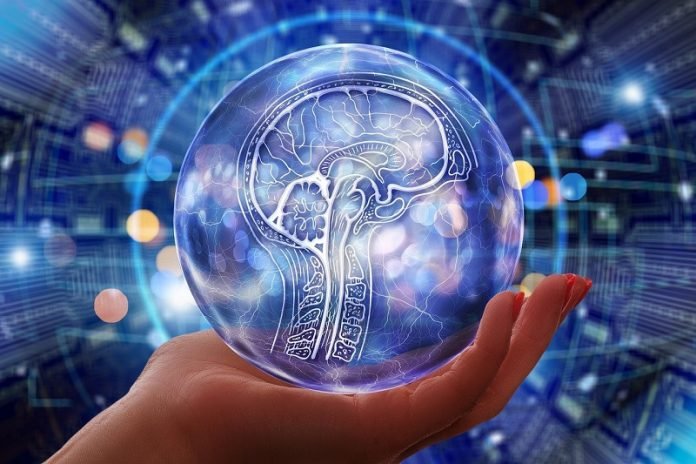
Since the advent of computing, people have lauded technology’s potential to act as a human brain.
In truth, computers have worked nothing at all like a brain for most of their history. In recent years, though, they’ve been getting closer.
In a new paper in Nature,“Towards Spike-based Machine Intelligence with Neuromorphic Computing,” Priyadarshini Panda and her co-authors Kaushik Roy and Akhilesh Jaiswal, both at Purdue University, provide an overview of the computer’s long and ongoing road to achieving something akin to the thinking power of the brain.
Panda, assistant professor of electrical engineering, emphasizes that there’s still nothing that acts like a brain – not least in part because much of how the brain works is still a mystery.
Plus, the brain remains a lot more powerful – there’s no silicon chip that can get close to the massive 3D connectivity of the brain.
That said, there are “bio-plausible” ways that computing technology can emulate both from algorithm and hardware perspectives to achieve brain-like ability with brain-like efficiency.
“It’s about being guided by brain-like principles,” she said.
“It isn’t biomimicking, because the brain does a lot of other things, but being guided by the brain is important – to be guided by the learning principles and the efficiencies that biology offers.”
One way to do that is through what’s known as spike-based machine learning. In the standard chip, transistors work all the time.
In the brain, though, neurons fire off – that is, exchange or transfer information – only when needed.
That’s known as event-driven spiking and it’s more energy-efficient, which translates to faster processing. On the hardware end, there is a lot of interesting research in beyond Silicon devices and beyond von Neumann architectures that can accelerate spike-based computing, and circumvent the limits of Moore’s Law, which states that chips will continually take on more transistors – a notion that experts believe is nearing its end.
“A lot of these semiconductor companies, like Intel and IBM and HP Labs, are heavily invested in this because they want to see the research in these next-generation devices and how they can empower the next generation of artificial intelligence and computing.”
As humans, she said, we reason very well and make decisions based on that reasoning. But today’s computing systems don’t yet have that capability because they don’t have the right kind of algorithms.
“We need to bring in those aspects to build truly functional, intelligent systems, which not only perceive the data around them but can also reason about it,” Panda said. “To be able to make decisions and reason about the data in real-time – that will be truly intelligent.”
There are formidable obstacles to getting there and overcoming them requires broad cooperation.
“It has to come from both a top-down and bottom-up approach – hardware has to understand what the algorithms require and the algorithms have to work within the constraints of the hardware,” she said.
Also, she said, it no longer makes sense for computers to have separate memory and processors. The traffic between the processor and memory is “the key bottleneck,” and it’s keeping computers from achieving the kind of energy-efficient synaptic learning possible in the brain.
Working with the neuroscience field, she said, should continue to prove valuable – for both fields of research.
“A lot of neuroscience people have been using deep learning models to decode some aspects of brain activity or fMRI data,” she said. “We’re looking at what the brain does and taking those abstractions from neuroscience and applying them to the computing field.
But we can also use these computing frameworks to help neuroscience better understand the learning capabilities of the brain. I think it’s going to be a two-way affair.”